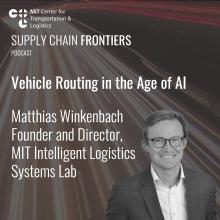
Vehicle routing is one of the most well-understood, extensively studied problems in both history and academia—it's been studied by academics since the early 1800s. Yet 200 years later, optimal efficiency remains just out of reach. And given the enormous increase in pressure for consumer expectations in recent years, that remaining "last mile" of vehicle routing efficiency could, on a global scale, make a huge difference to a huge number of people.
In this episode, we're joined by Matthias Winkenbach, Director of Research at the MIT Center for Transportation & Logistics. Matthias is an expert in urban logistics, last-mile delivery, and vehicle routing, and he has just launched a new lab, the MIT Intelligent Logistics Systems Lab, that will use AI and machine-learning techniques to tackle today's vehicle routing challenges—and that could make a major impact on vehicle routing solutions where traditional methods and algorithms have come up just short.
Transcript
- Before we get started with today's episode, we have some exciting news to share. We're pleased to welcome a new member to the MIT Global SCALE Network, the United Kingdom Supply Chain Excellence Center. This newest scale center has just launched through a partnership between the MIT Center for Transportation and Logistics and Loughborough University in the UK. The UK Supply Chain Excellence Center, based in Loughborough, will offer a master's degree program in supply chain management based on the curriculum developed at MIT and will soon begin admitting students to its inaugural cohort, the class of 2026. To learn more, visit scale.mit.edu. Welcome to MIT "Supply Chain Frontiers", presented by the Center for Transportation and Logistics. I'm your host, Benjy Kantor. Each episode of "Supply Chain Frontiers" features center researchers and staff or experts from industry for in-depth conversations about supply chain management, logistics, education, and beyond. Today, our guest is Dr. Matthias Winkenbach, a principal research scientist and newly appointed director of research at the MIT Center for Transportation and Logistics, as well as the director of the Megacity Logistics Lab, the Computational Analytics Visualization and Education Cave Lab. And soon, thanks to foundational support from and collaboration with Mecalux International, the newly launched Intelligent Logistics Systems Lab, all of which are under the purview of MIT CTL. First, this episode of MIT "Supply Chain Frontiers" is brought to you by the MITx MicroMasters® Program in Supply Chain Management. Gain an end-to-end understanding of supply chain management over five courses and a final comprehensive exam representing the equivalent of one semester of coursework at MIT. Boost your skills at work or pick up some knowledge as part of your lifelong learning. To learn more, visit micromasters.mit.edu/scm. Today, vehicle routing is one of the most studied, if not the most studied issue in logistics, stretching back about 100 years. But despite such attention and major strides in the decades since, optimal efficiency in vehicle routing and last-mile logistics remains just out of reach. But the comparatively small amount of ground that's left to cover stands on a global scale to have an enormous impact on supply chains, and AI and machine learning might be the keys to finding the remaining pieces of this puzzle. It's not as simple as getting from point A to point B, and Mathias Winkenbach is here as an expert in urban logistics, network design, data analytics, and more. And he's here today with us to discuss his very exciting work with using AI and machine learning to fully optimize vehicle routing and last-mile delivery. Matthias, welcome to "Supply Chain Frontiers". We're so happy to have you.
- Thanks, Benjy, for having me.
- Let's start with some context. Vehicle routing is one of the most studied problems in logistics, but also one whose solution is the most elusive. Can you give us a brief overview of, as best you can, the last 100 years or so of vehicle routing, or if you even can go that 100 years?
- Sure. I mean, the thing about the vehicle routing problem, and that's actually why we also focus our research on this with these new methods on the horizon, is that it is one of the most well-understood, well-studied problems in industry but also in academia. So if you look back, the foundational basis of vehicle routing problems is called the traveling salesman problem. That's a problem that academics have basically been studying since the early 1800s. The vehicle routing problem, which we today mostly know and study in the context of logistics and transportation, that was first formally introduced in 1959, I believe, by a researcher called Dantzig and Ramser. So it's been a while since we've been studying this problem, and so the state of the art in this space is also pretty advanced by now. So there are a lot of really good algorithms, models, to capture a lot of the real-world complexity that comes along with real-world vehicle routing problems, but the way they are solved today still struggles with basically getting the last, let's say, 20% right. And those last 20% didn't really matter that much for a long time, when we were in a world where people didn't shop online, and transportation did have enough time to basically get stuff from A to B, get stuff from a warehouse to a customer or a shop or a factory. But in the last couple of years, the industry has seen so much increase in pressure from consumer expectations. Things had to move faster, had to be more customer-centric, had to be more individualized. And suddenly, for these highly responsive logistics services that people are asking for today, those last 20% matter a lot. And that's where existing methods tend to reach the boundary of what they're capable of.
- Now, I first heard the term last mile with regard to people moving, commuting. I worked for and I was a member of a public bike-share system, and it was around 2014 or 15 or so. Is that the kind of thing you're looking at also, or is it specifically commercial, industrial, the things like Amazon getting your package to your door as opposed to getting you to work on time?
- I mean, there are certain similarities, but what makes last-mile logistics, so basically the commercial movement of goods on that last mile, particularly complex is that we are dealing with what we call fragmented routes. So if you are a commuter, you're usually an individual who has to get from point A to point B. And yes, you're making routing decisions along the way, but in a way you're just trying to find the shortest or most convenient or fastest path from point A to point B. While someone like Amazon, UPS, you name it, they have to deliver to multiple customers along one consolidated and efficient route, so think of the big brown van on the road out there. That van typically makes around 120 stops a day, and those have to be sequenced in a way that maybe you're not always able to take the shortest path between any two customers along that route, but your goal is to optimize the overall route. So to, for instance, find the overall fastest, cheapest, shortest path that connects all of these, in this case, 120 points on the map, basically.
- And when we're talking about the full efficiency of that last mile, and when I say efficiency I mean sort of all these things, like the cost and the speed and whatever the other factors are. Why has it been so elusive to get to that, the thing that makes it work completely?
- There's a few things that make this really hard. One is it's not just a math problem; it's a human problem because one major source of uncertainty in routing is, for instance, the driver. Whether the driver is very experienced or not, whether the driver is having a good day or a bad day that morning is also a factor of how other participants in a mobility system like a city, for instance, interact with that one route. So, for example, companies like UPS can optimize the heck out of a route, but then what happens if unexpectedly there's a traffic accident? And that completely changes your assumptions around travel speeds, travel times from one customer to the other. So there's a lot of uncertainty, a lot of factors that are very hard to control, if not impossible to control for the company when it tries to find the optimal solution. And that's where, let's say, traditional solution approaches to this problem tend to struggle, because they're either not capable of capturing all those uncertain influences, or they're not kind of efficient enough to quickly respond to changes in the operational environment to basically find the next best solution.
- And I know you've mentioned in the past that the complexities around this are based a lot in density. Now, if we're talking about density we're talking about population, are we talking about infrastructure? What are those things that are standing in the way that are challenging, or that create the problems in the first place that aren't necessarily standing in the way but they exist, and so you have to figure it out?
- So, I mean, there's a reason why that one lab that I've been leading for the last 10 years roughly is called the Megacity Logistics Lab, Because when we started out doing this research we very much focused on really large cities. Why? Because that's where a lot of people live But, also, that's where logistics processes are the most complex. And one of the main drivers of that complexity is indeed density, So every city, or let's say most cities out there weren't planned for the size and the amount of people and the amount of goods that need to move into and out of these cities today. Most cities out there grew historically, and that's okay but the problem is that usually transportation infrastructure doesn't grow proportionally, because it was never designed to really be able to deal with that much traffic, that much demand for this limited space on the road, basically. And that's where, as cities grow, as we see ongoing urbanization all around the world, density in these cities increases and that means traffic density increases, demand density increases. And that, again, has both positive and also negative effects on logistics processes. The positive thing is, if your customers are more densely co-located, then at least theoretically you have to travel less to meet the same number of people and to deliver the same amount of goods, for instance. So technically, your delivery routes should get shorter, faster, cheaper. But the downside of it is, as I said before, transportation infrastructure didn't grow proportionally so you have more traffic, more uncertainty, generally mobility decreases, travel speeds decrease, so that makes these routes not just less efficient but also less predictable because there's a higher likelihood of a random incident like a traffic accident in a high-density environment as compared to, let's say, a low-density environment like rural Massachusetts.
- I'm glad you brought up the Megacity Logistics Lab. I'm kind of curious, can you tell us how that lab fits into what the current methods are, what the new methods are, considering it's only 10 years, it's only been around for 10% of the time that this problem has been focused on. And so what are the new methods for looking at these older problems, and how do they differ from what people were trying to do before?
- Well, I mean, when I started researching this space, which was shortly before the Megacity Lab started, but that was basically at the onset of widespread e-commerce. That's when Amazon just started operating, basically. That's when people kind of did realize how important the last mile of any logistics process might become in the future, but probably nobody really had it on their radar that today we're talking about services like order now and get it within the next two hours or something like that. And so the traditional methods that also my lab predominantly has been using over the last 10 years can be generally classified as what we call operations research methods. So this, broadly speaking, means optimization methods. And here we mostly distinguish between exact methods, so these are typically mathematical models where you formulate, let's say, a routing problem exactly. So you write it down as a set of equations and then you're using algorithms to solve those equations exactly. So, ideally, at the end of the day you want to get the optimal solution. The problem about these methods is that as soon as you apply them to real-world problems that are of a real-world size, like for instance those 120 stops of the big brown van that I mentioned earlier, those methods take a very long time to find that optimal solution, if they can find it at all. So they're not efficient enough to really be applied at scale, typically. That's why the other family of methods that fall under kind of traditional operations research would be heuristics and meta heuristics approaches, which is basically a set of rules, a set of kind of decision procedures that you put into an algorithm that are often tailored towards the specifics of the problem. So for instance, in the context of vehicle routing, you can encode certain rules that define what is a good or a not so good move of a vehicle from one customer to the next based on your knowledge of the problem, based on your domain expertise. So these methods tend to be faster, tend to be more efficient in finding really good solutions. The downside is that they typically can never guarantee that they find the optimal solution. So that's just kind of a two-minute summary of what those traditional methods are. And my lab has been very active in publishing papers, working with industry on using both of these types of methods extensively on solving all sorts of last-mile logistics problems, not just routing. So the reason why we are now exploring a new set of methods inspired by all this generative AI and machine learning stuff that's going on out there today is that, as I said before, the world is moving faster than these traditional methods can keep up. So for instance, being able to optimize the logistics system for services like on-demand one-hour delivery means you are optimizing a route with very little time at hand. And then some of these existing methods, some of these traditional methods might just not be fast or efficient enough to really solve your problem within that little time. Similarly, the trend towards more customer centricity, so really focusing logistics processes around the individual requests, the individual needs of individual customers, exposes them to so much uncertainty that, again, these traditional methods have a hard time capturing all of that, capturing the uncertainty and the real-world complexity of, let's say, a modern-age state-of-the-art routing problem. And that's where we need approaches that are more data-driven, that learn more from real-world operational data, that learn more about the real characteristics of individual customers or individual drivers, or individual routes. And these data-driven methods can't really be found in the traditional operations research space, but are very prevalent in what is currently being discussed as machine learning and AI methods.
- I'm really glad you brought up that idea of needs and how efficiently someone might need to have a package delivered to them, or how quickly a company needs to have delivered. And I'm gonna come up with a more challenging question for later in the show, but something else that you touched on is as you are working more and more with AI, machine learning, and predictive analytics, how is that AI differ from simply someone or a group of people analyzing data that they already know exists, and making a prediction based on that?
- That's the thing, I mean, there's been research on predictive analytics for decades. And I'm also not saying that this will become irrelevant in the future, but to give you a very tangible example, if you're looking at demand forecasting today, there are very capable methods to forecast, let's say, the demand for a certain product in, let's just say, Amazon's assortment at the scale of an entire city for maybe the next day or the next week. So you can probably relatively accurately predict how frequently that product will be bought in the city of Boston next week. But if we're talking about customer-centric logistics processes with very short lead time, let's stick to the example of on-demand one-hour delivery. Being able to predict how often that product will be bought next week is pretty much useless for you, what you really need is the ability to predict at a very high spatial but also temporal resolution when and where that product is going to be bought. So basically, what you need to be able to do is to have a good estimate of how many items of that product will be bought, let's say, in a zip code in Cambridge within the next 30 minutes. And that's where those traditional methods that rely on a lot of data to make kind of point estimates or distribution estimates are not that useful anymore, because you need tools that take much more data into account but also detect much more interdependencies between the various data points that you use to train these models, to be able to make these more fine-grain predictions. That's one difference between what used to be just prediction versus what we now call machine learning-based predictive analytics. But the other thing that I would like to point out, and that brings us back to routing, is, for instance, up until recently, predicting a really good vehicle route was not something that was considered really feasible because, let's say, if you are to predict a good solution to a routing problem, you're not just making one point prediction, you're not just predicting one value far out in the future, you're basically predicting a solution to a sequential decision problem. So you're predicting a sequence of stops of a vehicle that has to make sense. Not just one stop that has to make sense, the whole sequence of stops has to make sense, it has to be a solution that's both feasible, that's even viable to execute on, and at the same time should be as close to an optimal solution as possible. And that's something where, let's say, traditional prediction methods were just not capable of doing that. Now, with the advent of stuff like transformer models, so actually more or less the same kind of methods that also power things like ChatGPT and all of these chatbots, even though they are using these methods in, let's say, the context of natural language processing, these types of methods also have a huge potential for optimization problems in the logistics industry, like the vehicle routing problem.
- Something funny that came up recently in a conversation I was having with Jose Velasquez, with regard to some Amazon delivery and last-mile stuff was that some of the things that seem very intuitive, for instance, that you might think that it is most efficient to deliver packages in a way that it has the best geographical routing, but actually that might not be the most efficient in terms of sustainability, and even speed, because maybe it has to do with how heavy the packages are and things like that.
- So another example in that space is, and something that we also explored a few years ago already with folks like Amazon and others, is you wanna come up with route plans that are not just short or cheap or fast, but that a driver can actually execute on and that a driver actually thinks is a good route. And here's one of the big benefits of these more data-driven learning-based approaches as compared to just running an optimization model, because a driver, especially an experienced one, knows exactly how to operate a route. And if you look at many drivers out there today, they get a route plan in the morning and they make their own adjustments because, for instance, they know much better than any routing algorithms where to park safely, where to basically avoid traffic at certain times of the day, or they might even know which customer might be more or less available during which times of the day, even though that customer may have never explicitly voiced a preference for, let's say, being delivered between 10 and 11 AM, but the driver knows this. And that kind of consistency, that kind of tacit knowledge that's ingrained in every single driver, is basically impossible to encode in a hard optimization algorithm or a set of mathematical equations. But it can be learned from observing the actual behavior of drivers over time, so we may not necessarily come up with shorter or faster or cheaper routes, but we may come up with routes that are more reliable because the driver is actually able to act on the route plan that he gets in the morning, or she, and also some of his or her tacit knowledge about that route is already embedded in the plan.
- And is that gonna change or has it been changing the more there is autonomous delivery, or drone delivery or things like that?
- I mean, today, for better or for worse, there is not that much autonomous delivery yet, especially not in urban contexts but let's say if we were ever to get to that point, what you wouldn't wanna lose if you were fully automating the delivery process is that tacit knowledge about, for instance, individual customers or safe and available parking spaces and the like. And so you would wanna have some sort of trained model running in the background that tells that autonomous vehicle, or drone or whatever it might be, how to best deliver the product to your customer, even though there is no human involved anymore. So in a way, if we want these systems to be as close to a human delivery experience as possible, then sure, this is the kind of method that we should explore further.
- And when we're talking about route optimization, have you seen trends that companies are prioritizing when they're optimizing their routes? Have you seen those shift from the past? Are there things that your teams are working on that you've seen start to be implemented, and how is that changing the landscape?
- I mean, I think that the underlying routing problem, I mean, as I said, that has been studied for decades. The fundamentals of that problem don't necessarily change, but what has definitely been a trend is how can we focus our solutions to the routing problem more around the specific needs of individual customers? So, while a few years ago you treated every single customer more or less as a uniform mass, now it's really more about individual requests and individual characteristics. And that obviously also has implications for the way companies approach these problems. They have to capture more stop-specific information, for instance, how much does it actually take me to deliver to customer A versus customer B? And that may depend on the nature of that customer, the nature of the building that the customer is in, and many other factors. But, also, we need to understand preferences more explicitly. And a few years ago people would opt for something like time window delivery, and you would get immediate input from your customer as to when they wanna be delivered to. Nowadays, the expectation is more like, "Well, the logistics process should already anticipate my availability, my preferences." So even though I don't voice these preferences explicitly, your routing algorithm should probably already take into account what we have learned about that customer over time, and therefore basically make that customer happier by, under the hood, already customizing the delivery to his or her preferences. So that shift towards more customer centricity comes in various shapes and forms but has definitely been the most important driver of complexity, but also innovation in the routing space.
- I'll bring in my more challenging question here, which is talking about customer preferences. If I order a soccer ball online, my preference would be that my soccer ball's in my hand right now. How do, I guess, we balance the, there's one part that's just sort of like... Again, the logistics of getting that into somebody's hand as fast as they want it versus as fast as they need it, I guess, is the question, which is like the prioritization when it comes to- You know there's labs at CTL that deal with sustainability and humanitarian, and if delivering that soccer ball is gonna impede my delivering water or baby formula or something like that. Is that sort of, for lack of a better term, morality in decision included in how machines learn, how people look at it, how companies put that into effect?
- Let's say it could be, but it's something that, let's say, we haven't explored the morality of vehicle routing problem explicitly yet because that's honestly a rather subjective term. It comes down to the same problem that many of those autonomous vehicle manufacturers have today, like, how do we teach a vehicle morality if it has a choice between, I don't know, having an accident that, let's say, kills a child versus kills an old lady? What's better, what's worse? We don't know. And as humans, if there's a human driver, we usually don't really have a choice because it just happens so fast. A machine might actually have a choice, so how do we teach it ethical behavior? And that's something that we are probably not equipped to do in our lab, and that's why we try to stay away from that. But what you can do, obviously, as a company is you can prioritize certain objectives in your decision-making. So for instance, if it's maybe not a life-and-death decision, but if it's about reducing your overall emissions footprint as a whole, as a company, let's say, if an e-commerce platform wants to make sure that it still meets the customer requirements as good as it possibly can, but at the same time tries to be as sustainable as possible, then that may be part of that rather complex set of objectives that you can "teach" to a routing algorithm so that that algorithm probably already makes that distinction on a product level and say, "Okay, if I have to deprioritize certain products because either I run out of capacity or because I'm hitting my emissions threshold, basically, then which one should I deprioritize, the soccer ball or the baby formula?" And so in a way, that's another opportunity, I believe, where these algorithms also have a unique strength, which is learning from customer feedback. If you get your soccer ball a few hours or maybe a day late, you might not be thrilled about it but it's also not the end of the world. If the mother of a newborn child runs out of baby formula because the delivery is getting late, she will probably be much less thrilled. And if you can capture that feedback somehow and basically use that as your input, from which you can then learn or train an algorithm to kind of check its priorities a little bit, then next time you have that problem that algorithm is already better equipped to anticipate the kind of discomfort or disutility that it is causing by deprioritizing the wrong thing. So in that sense, rather than you and I who design an algorithm having to make an explicit choice, whether it's the formula or the soccer ball, we can actually have those algorithms learn from the response of the entire kind of social system, which is, in this case, your customers, to your priorities. And over time, those would then hopefully match. So what your algorithm does matches both your corporate objectives, as well as the general norm or the general priorities of your customer base.
- Yeah, I appreciate that. I don't know that the question has an answer, just kind of curious when talking about the different sort of research aspects, especially within CTL, just thinking about those kind of priorities and what kind of goes into this. Shifting gears a little bit, I would love to hear you talk about this new venture, this Intelligent Logistics Systems Lab, where the research goes from here. Is this a step farther from what the other labs that you're directing are doing, or is it a completely different type of research?
- I mean, the formation of the Intelligent Logistics Systems Lab is basically born out of an observation that we've had for several years now, namely that more and more of the research that my other labs at CTL, but also other labs at the center and other places at MIT, are doing, more and more of that work moves away from basically those traditional methods that we discussed before, those traditional operations research methods, and move further towards this "new family of methods" in the machine learning and AI space, and most importantly we see it as a huge potential for work kind of at the intersection of the two, basically hybrid methods that basically try to combine the best of both worlds, the best of traditional OR methods and the best that those new ML and AI methods can offer. Because what we see in our early research already today is that actually the greatest potential for improvement over what we've been doing for the last couple of decades doesn't necessarily lie in completely replacing those methods, but rather kind of putting them on machine-learning steroids, if you allow that term. And that's kind of an exciting observation for us and also kind of goes beyond the original scope of, for instance, the Megacity Logistics Lab and many other labs at the center. So I think it's the right time to actually have a dedicated group at the center that bundles our activities in that space a little bit more, and really envisions kind of the future generation of logistics systems that enable logistics services that we probably today can just kind of imagine, but that will have to become a reality within the next couple of years, because that trend towards further customer centricity, increasing pressure of sustainability, increasing needs for supply chain resilience, all of these trends require us to build systems that are smarter in the sense that they are more agile in responding to changes in the operational environment, in the socioeconomic environment, in the geopolitical environment, and that are more data-driven. That are basically, as I said before, learn from the very rich knowledge that exists in many individuals out there in the logistics industry, be that the driver, be that the warehouse worker, be that the supply chain logistics executive with 40 years of industry experience. We don't wanna lose the human expertise in designing those future systems, but we need to be able to scale that expertise and basically ingrain it in the mathematical models that we build, such that we can solve these very challenging future logistics systems design problems at scale and rapidly.
- This is something that I've asked a few of your colleagues about their labs. Is there an end goal for the lab? Is there a point at which you have done what you need to do, you've antiquated the lab in a good way because the solution has been found?
- Yeah, I mean, in a way the Megacity Logistics Lab might be a good example for that because it's been around for a bit more than 10 years now. We've done a lot of great work but the lab has to some extent outgrown itself, so we no longer just focus on last mile logistics in the very narrow sense, we are basically already working on a lot of stuff that involves more than just the last mile, because today the way you operate a last mile vehicle route isn't a problem that is isolated from everything else in the supply chain, it's basically highly interconnected with aspects of network design, inventory, planning, sourcing decisions, long-haul transportation decisions. So supply chains and logistic systems are, or have become much more interconnected so, basically, focusing on just that one aspect of last mile logistics may no longer make that much sense, at least in many cases. Which is one of the reasons why, I mean, the lab will still exist but I think our focus will probably shift towards looking at supply chains and logistics systems at a slightly higher level, which is in part enabled by also looking at a broader set of methods to basically not just optimize that single vehicle route, but ideally to find an optimal solution to an entire system that may consist of many different vehicle routes, 1,000s of customers, large networks, many interconnected decisions. And ideally we want to find ways to solve these problems in an integrated way, so just focusing on last mile in this case might not necessarily be what we need to do to design the next generation of logistics systems.
- We talked a little bit earlier about sort of the satisfaction for an end consumer. What are the implications for everyday consumers? Again, we talked a little bit like delivery of the soccer ball, but...
- I mean, the obvious benefit to the, let's say, the end consumer or people like you and me in their day-to-day lives are what we already discussed. So we are seeing today that we get kind of a level of quality and speed of delivery services that would've been unimaginable a couple of years ago. So today, at least if you live in a major city, you can literally go online if you run out of toilet paper, if you wish, and get it delivered the same day or, at worst case, the next day if you really need it. So that level of service and convenience is, it's not gonna stop there, there's gonna be further advancements in this space. So just customer utility is going to continue to grow based on these innovations in the logistics space, but that's honestly not just the only thing that we care about. The other very big benefit that may be a little bit less obvious is sustainability, and that affects every single human being on this planet, given what we've been seeing over the last couple of years in terms of climate change and the like. The logistics and transportation industry is one of the main contributors to carbon emissions, and therefore to the things that eventually cause climate change. And if we don't manage to decarbonize this industry, we will not be able to stop it or to even slow it down. And so one of the reasons why we care deeply about using these new sets of methods to design better future logistic systems is that, at least my personal belief is that without these types of methods, without these types of innovations that we seek to develop in the new lab, we will just not be able to meet the targets that the industry has set itself in terms of rapid decarbonization. And the faster we can decarbonize this industry, the better for everyone. But with traditional methods, there's just a limit to how much we can squeeze out of the system, because it is a very, very hard to decarbonize industry so you really need to have the smartest possible algorithms and models in place to make your existing logistics systems, and especially your future logistics systems, as efficient as possible. So that's one major kind of driver of value, I believe, to anyone on this planet, even people who may never consume these logistics services. And last but not least, I mean, we talked a lot about cities, my other lab, the Megacity Logistics Lab, is focused on urban logistics but a large part of this planet's population is still not living in cities, and also many people on our planet cannot afford things like same day delivery. And basically making those high-end logistics services more accessible to a broader part of the population, to other sociodemographic kind of groups within our society, but also to people who just do not live in major urban centers, that is another goal, I believe, of our lab, to basically not create kind of two separate worlds in terms of logistics service and logistics innovation, but ideally to make those innovations accessible to as many people as possible.
- You've mentioned the different kind of things that create value for the end consumer, and you've told me this, correct, that last mile delivery represents 40% of total logistic costs for companies. So I can imagine from that economic standpoint that looking at that is gonna be a value for the company, is there anything even close that companies are dealing with that are close to that 40% of logistic cost, or is that really the top?
- Obviously it depends a little bit on which industry you're in, what product you're building and selling, but on average that's a pretty good proxy, like 40%. And I mean, think of, I don't know, the clothes we're wearing today, they've probably been shipped around the world at least once, and still that last mile, so really just getting it to you or to the store where you bought it from one of the big warehouses nearby.
- Well, I sew my own so I'm . All self-made, no, just-
- In that case you will have to come up with the cost but, in general, like that last mile, that shortest little piece of the supply chain is really still by far the biggest contributor to overall supply chain cost. And that's also one of the reasons why, even though problems like the vehicle routing problem have been tackled for decades, it's still worth trying to solve them in an even better way because even small little improvements in the way we execute the last mile have tremendous impacts on our cost basis. So, yeah, in that sense it is still one of the main kind of also economic drivers of success for a company that's supply chain driven. And that eventually also boils down into the cost that a consumer has to pay to, for instance, receive a certain product.
- I imagine, also, that last mile accounts for a pretty good portion of the emissions that are, vehicle emissions coming out as well. I just hope you would speak to that a bit.
- Yeah, so it's probably important to distinguish between kind of the emissions footprint that is caused by last mile logistics directly versus indirectly. So if you think of last mile logistics as that last delivery route of one of those big brown, yellow, white vans out there, sure they are creating emissions but, let's say, in the grand scheme of things if you look at greenhouse gas emissions, they might not play such an important role. So electrifying, for instance, those last mile fleets is still important, but it will probably not necessarily turn the needle when it comes to avoiding or slowing down climate change. Those last mile delivery routes are, however, for instance, a major contributor to pollutant emissions that have immediate health impacts. Think of particulate matter and NOX and the like. So that's actually one of the more immediate effects because especially in an urban environment where you have a lot of people, a lot of traffic, a lot of these commercial vehicles operating in a kind of close space, those immediate health and other kind of related aspects, those immediate health impacts are very measurable and one major reason for companies to seek to replace those fleets by less polluting technologies. But probably the more important thing to consider from a greenhouse gas emissions point of view is actually the indirect emissions caused by last mile logistics. So imagine a company who starts offering things like same day, one hour, on demand delivery. Sure you have a last mile delivery route that needs to get that product once it's ordered from, let's say, the store to the customer, and that does have emissions, but the much bigger emissions impact is actually related to the question how the product gets to that store in the first place. Because suddenly you are operating out of a network where you're not fulfilling all your customer orders out of a network of only a few really big, highly consolidated warehouses or distribution centers, but instead you are actually operating out of a highly fragmented network of stores, micro-fulfillment centers, whatever you might call it. So you have to move stuff around your network much more frequently and in a much more fragmented way, and that creates basically more miles driven per package, if you wanna call it that way, and therefore also much more emissions per delivery, basically further upstream your distribution system. It's one of the reasons why focusing on last mile alone when optimizing a logistics process for something like sustainability emissions is probably missing the point. So you really have to think about these services at a systemic level. So, sure, you still have to solve that last mile problem, but especially when it comes to emissions and the like, you also have to consider the entire upstream process that gets the product close enough to the customer in the first place to even offer something like a one-hour delivery service. And that's why this is so hard to decarbonize, because some of these transportation activities that happen in between require you to overcome large distances by truck or, worst case, by plane or something like that. And these are just, at least currently, transportation modes and transportation lanes that are very hard to decarbonize from a technological point of view. So obviously there's a lot of research, and we are not involved in that, that tries to make, I don't know, planes and trucks more sustainable, less polluting, but at the end of the day what we care about is how do we avoid unnecessary transportation in the first place? And that brings us back to talking about, for instance, machine learning methods to help us anticipate customer demand better, because if we already can anticipate that customer A is going to order this product within the next two hours and he or she will want it within an hour, then that puts us in a position where we can pre-position the corresponding inventory, so we can basically move the item that he or she is going to buy to a place close to that customer already early on. And that, again, allows us to consolidate those transportation flows more effectively, and therefore reduce the number of vehicles, reduce the number of miles driven to basically achieve the same level of service.
- Is there anything you wish I was asking that I haven't? Anything I should have been asking that folks might wanna know about?
- A lot of people, I think, especially as we talk about AI and machine learning, are still struggling a little bit to see the true potential of these methods. And I'm not saying that we have figured it out, I'm not saying that I can really speak to the true potential, but let's say one of the reasons why I'm particularly grateful to Mecalux for giving us this generous seed funding for the new lab is that with them we found a partner who actually believes in the significant potential of these methods because, let's face it, we are only at the beginning of a journey here. So we have to do a lot of the groundwork on the research side first before we will be able to reap the full potential of these methods. That means that maybe for the first couple of, hopefully in a couple, but for the first few years at least of the existence of this new lab, we will make significant advances on the research side, but it might take a little while until we can turn this into tools and methods that can be scaled to industrial scales, and therefore basically have an immediate ROI for companies who wish to kind of employ these types of tools and methods. And basically finding someone who trusts in our ability to eventually get there and who gives us the runway to explore the fundamentals of this space, build the foundation of this new lab in the right way is what's really critical for us to make this lab a success. So I'm particularly excited about this piece, really trying to understand the fundamental building blocks of what will shape the future research of this lab with the support of Mecalux, and hopefully many other sponsors as well. But at the same time, I obviously wanna bring this to a point where we can really solve real-world problems at an industrial scale as quickly as possible because, as I said before, the problems that we're trying to solve aren't necessarily waiting on us. So climate change will not wait until we've figured out machine learning, but it's there, it's moving rapidly and we need to catch up quickly in order to have a chance to fix it. That's obviously a little bit daunting but at the same time exciting to embark on that new journey, and I'm sure with the students and the research team that I have, or that we all have at the center, we are more than well-equipped to be successful at this, so let's get started.
- Our guest today has been Dr. Matthias Winkenbach who holds myriad titles, including Director of Research at the MIT Center for Transportation and Logistics, Director of the Megacity Logistics Lab, as well as the CAVE Lab, and Director of the new Intelligent Logistics Systems Lab. Matthias, it's been a pleasure having you with us. Thanks for joining us on "Supply Chain Frontiers".
- Thank you for having me.
- Thanks for listening to this episode of "MIT Supply Chain Frontiers", presented by the MIT Center for Transportation and Logistics. To check out other episodes of "MIT Supply Chain Frontiers", visit ctl.mit.edu/podcasts. And for more on the center's research, outreach, and education initiatives, make sure to visit us online at ctl.mit.edu. Our producer is Dan McCool of the MIT Center for Transportation and Logistics. Our sound editor is Dave Lishansky of David Benjamin Sound, and our audio engineer today is Kurt Schneider of MIT Audio Visual Services. "MIT Supply Chain Frontiers" is recorded on the MIT campus in Cambridge, Massachusetts. Until next time, this is Benjy Kantor bringing you the latest insights from the leading edge of supply chain management on "MIT Supply Chain Frontiers".